New Research Reveals Limitations of Large Language Models in Multi-Turn Conversations
A recent study from Microsoft Research and Salesforce highlights a critical limitation in even the most advanced Large Language Models (LLMs): their performance significantly deteriorates when instructions are given in stages rather than all at once. The research found an average performance drop of 39% across six tasks when prompts are split over multiple turns:
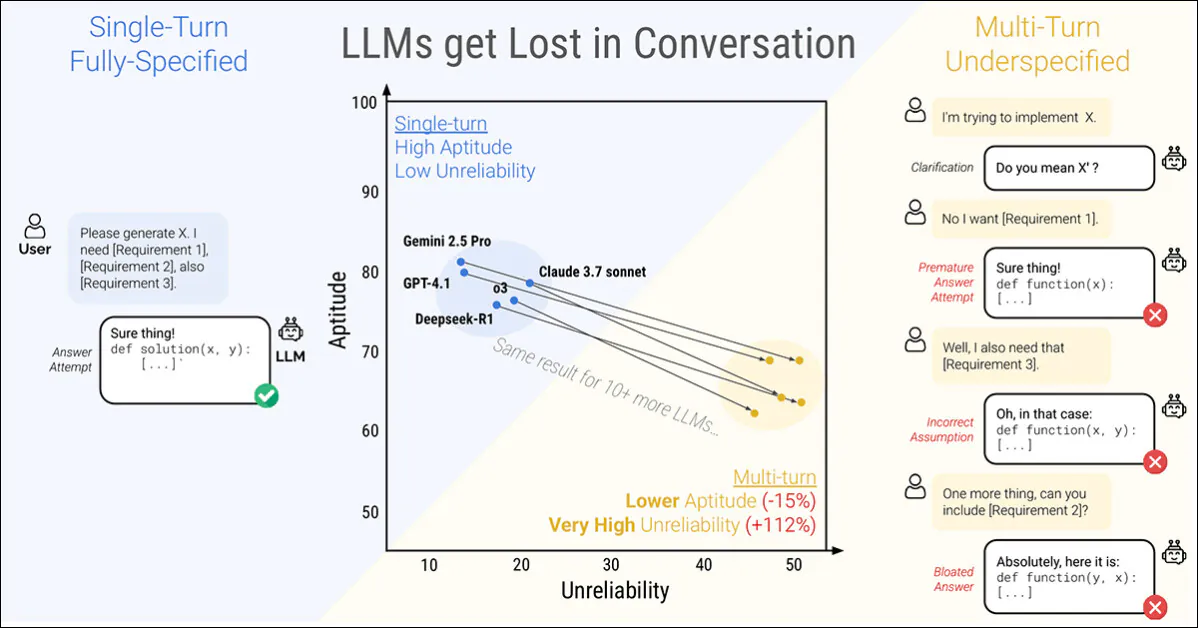
A single-turn conversation (left) yields optimal results while multi-turn interactions (right) lead to diminished effectiveness, even in top models. Source: arXiv
The study reveals that the reliability of responses drastically declines with stage-based instructions. Noteworthy models like ChatGPT-4.1 and Gemini 2.5 Pro exhibit fluctuations between near-perfect answers and significant failures depending on the phrasing of tasks, with output consistency dropping by over 50%.
Understanding the Problem: The Sharding Method
The paper presents a novel approach termed sharding, which divides comprehensive prompts into smaller fragments, presenting them one at a time throughout the conversation.
This methodology can be likened to placing a complete order at a restaurant versus engaging in a collaborative dialogue with the waiter:
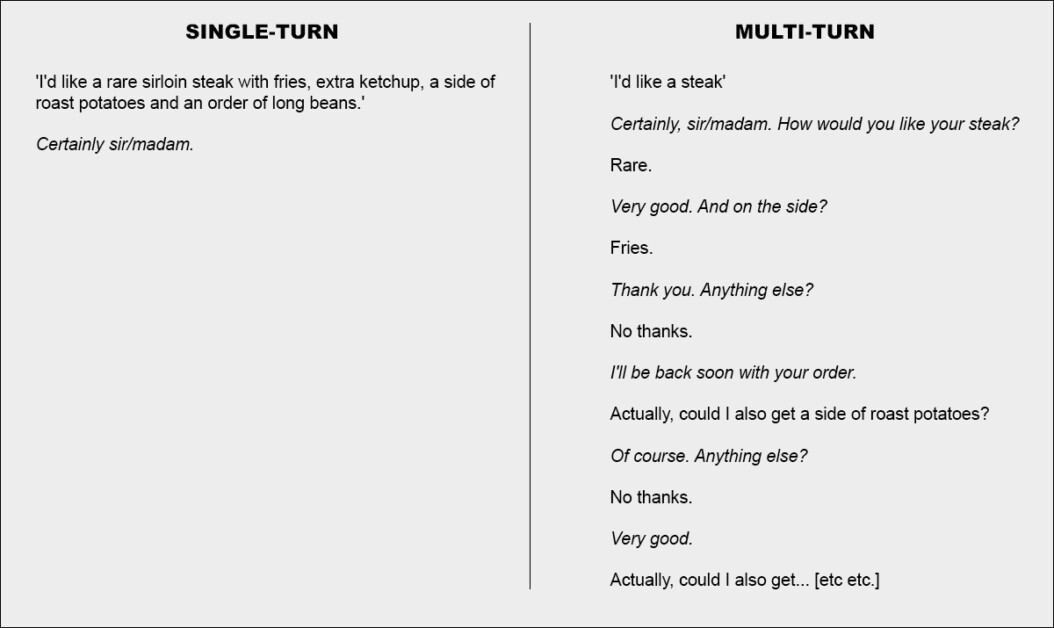
Two extremes of conversation depicted through a restaurant scenario (illustrative purposes only).
Key Findings and Recommendations
The research indicates that LLMs tend to generate excessively long responses, clinging to misconceived insights even after their inaccuracies are evident. This behavior can lead the system to completely lose track of the conversation.
Interestingly, it has been noted, as many users have experienced, that starting a new conversation often proves to be a more effective strategy than continuing an ongoing one.
‘If a conversation with an LLM did not yield expected outcomes, collecting the same information in a new conversation can lead to vastly improved results.’
Agent Frameworks: A Double-Edged Sword
While systems like Autogen or LangChain may enhance outcomes by acting as intermediary layers between users and LLMs, the authors argue that such abstractions should not be necessary. They propose:
‘Multi-turn capabilities could be integrated directly into LLMs instead of relegated to external frameworks.’
Sharded Conversations: Experimental Setup
The study introduces the idea of breaking traditional single-turn instructions into smaller, context-driven shards. This new construct simulates dynamic, exploratory engagement patterns similar to those found in systems like ChatGPT or Google Gemini.
The simulation progresses through three entities: the assistant, the evaluated model; the user, who reveals shards; and the system, which monitors and rates the interaction. This configuration mimics real-world dialogue by allowing flexibility in how the conversation unfolds.
Insightful Simulation Scenarios
The researchers employed five distinct simulations to scrutinize model behavior under various conditions:
- Full: The model receives the entire instruction in a single turn.
- Sharded: The instruction is divided and provided across multiple turns.
- Concat: Shards are consolidated into a list, removing their conversational structure.
- Recap: All previous shards are reiterated at the end for context before a final answer.
- Snowball: Every turn restates all prior shards for increased context visibility.
Evaluation: Tasks and Metrics
Six generation tasks were employed, including code generation and Text-to-SQL prompts from established datasets. Performance was gauged using three metrics: average performance, aptitude, and unreliability.
Contenders and Results
Fifteen models were evaluated, revealing that all showed performance degradation in simulated multi-turn settings, coining this phenomenon as Lost in Conversation. The study emphasizes that higher performance models struggled similarly, dispelling the assumption that superior models would maintain better reliability.
Conclusions and Implications
The findings underscore that exceptional single-turn performance does not equate to multi-turn reliability. This raises concerns about the real-world readiness of LLMs, urging caution against dependency on simplified benchmarks that overlook the complexities of fragmented interactions.
The authors conclude with a call to treat multi-turn ability as a fundamental skill of LLMs—one that should be prioritized instead of externalized into frameworks:
‘The degradation observed in experiments is a probable underestimation of LLM unreliability in practical applications.’
Here are five FAQs based on the topic "Why Language Models Get ‘Lost’ in Conversation":
FAQ 1: What does it mean for a language model to get ‘lost’ in conversation?
Answer: When a language model gets ‘lost’ in conversation, it fails to maintain context or coherence, leading to responses that are irrelevant or off-topic. This often occurs when the dialogue is lengthy or when it involves complex topics.
FAQ 2: What are common reasons for language models losing track in conversations?
Answer: Common reasons include:
- Contextual Limitations: Models may not remember prior parts of the dialogue.
- Ambiguity: Vague or unclear questions can lead to misinterpretation.
- Complexity: Multistep reasoning or nuanced topics can confuse models.
FAQ 3: How can users help language models stay on track during conversations?
Answer: Users can:
- Be Clear and Specific: Provide clear questions or context to guide the model.
- Reinforce Context: Regularly remind the model of previous points in the conversation.
- Limit Complexity: Break down complex subjects into simpler, digestible questions.
FAQ 4: Are there improvements being made to help language models maintain context better?
Answer: Yes, ongoing research focuses on enhancing context tracking in language models. Techniques include improved memory mechanisms, larger contexts for processing dialogue, and better algorithms for understanding user intent.
FAQ 5: What should I do if a language model responds inappropriately or seems confused?
Answer: If a language model seems confused, you can:
- Rephrase Your Question: Try stating your question differently.
- Provide Additional Context: Offering more information may help clarify your intent.
- Redirect the Conversation: Shift to a new topic if the model is persistently off-track.